Can a non-technical person become a data scientist?
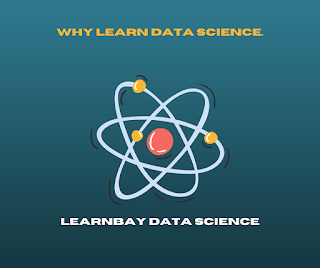
In 2020, the global revenues for big data and business analytics are set to reach $210 billion.
Naturally, because of increased demand, there has been a shortage of
data science professionals and firms are reaching out with open arms to those looking to embrace the
industry and join the workforce.
Naturally, because of increased demand, there has been a shortage of
data science professionals and firms are reaching out with open arms to those looking to embrace the
industry and join the workforce.
Any person with a structural thought process, good logical thinking skills, conviction towards learning
new tools and with a good business perspective can get into the field of data sciences.
new tools and with a good business perspective can get into the field of data sciences.
Those looking to close the gap and jump from a non-technical background to data science and related fields,
do not fret! These tips will guide you on how to get a foot in the door and stay at the top of recruiter’s minds
when new and competitive job opportunities pop up.
do not fret! These tips will guide you on how to get a foot in the door and stay at the top of recruiter’s minds
when new and competitive job opportunities pop up.
Here’s how to get into data science from a non-technical background:
Identify your dream job
In Technical Jobs Most Roles Require these Skills
-
Mathematics
-
Statistics
-
Programming
-
Business knowledge
Mathematics
Statistics
Programming
Business knowledge
and experts from other fields.The long-term aim of data science in the context of business is to derive
insights that can drive business planning and future goals like increasing revenue, powering sales
and recruiting top talent. However, the technical skills that would aid in your dream
job are decided only when you understand which path you want to follow.
Another crucial step is evaluating what current knowledge is applicable within
this field, despite being sourced from another field.
This is especially true of graduates in economics, mathematics, statistics or business
studies– facets of these streams are well-embedded in data science, so make use of them.
The first step to learning is knowing what you need to learn!
this field, despite being sourced from another field.
This is especially true of graduates in economics, mathematics, statistics or business
studies– facets of these streams are well-embedded in data science, so make use of them.
The first step to learning is knowing what you need to learn!
Learn new skills through a data science course
Data science is a complex field interwoven with facets of different industries.
To be able to get a head start in the field as a novice with little to no knowledge,
candidates must up skill by enrolling in a well-outlined course with top educational institutions or
course providers.
The ideal curriculum should cover the following topics:
To be able to get a head start in the field as a novice with little to no knowledge,
candidates must up skill by enrolling in a well-outlined course with top educational institutions or
course providers.
The ideal curriculum should cover the following topics:
Deep learning
Data visualization
Statistics and probability
Big data handling
List of the top 5 must-have technical skills for a data scientist.
- Programming
A data scientist will be proficient in one of the programming languages like R, Python, SAS, Hadoop, etc. It is not just about writing code but being comfortable with using different programming environments to analyze data. With the field of data science seeing unprecedented interest and value in businesses around the world, a command over programming languages and the ability to adapt to the changing technology is key to a data scientist’s success. Any hesitation with using programming tools can turn into a deal breaker for a company relying on your work to accelerate their business growth. - Quantitative Analysis
This is what defines the essence of a data scientist’s job. Having a calculated and visceral understanding of a complex environment and its behavior, munging data that is messy and difficult to work with, creating prototypes and models to test assumptions is part of a data scientist’s profile. Must-know concepts include how to build predictive and regression models, machine learning – supervised and unsupervised learning algorithms, time-series forecasting, data-reduction techniques, neural networks, etc. - Statistical Knowledge
Without statistics, a data scientist and the future of an organization is at sea. Generating hypothesis based on how a system will behave with changes, making assumptions of statistical significance about variations in data, defining metrics to layout objectives and measuring success, and drawing accurate conclusions from the dataset will all be impossible without math and statistics. Writing code or using functions effectively will also become a challenge if a strong foundation in math and statistics is missing. - Visualization Skills
It’s a known fact that humans absorb information faster in the form of pictures as compared to words and numbers. A working knowledge of data visualization tools like Tableau, Qlikview, Plotly, or Sisense will ensure that a data scientist is confidently able to present insights to both a technical and non-technical audience convincing them of the business value their insights can draw. Acquainting themselves with the principles of visualizing and presenting compelling data to stakeholders can go a long way in determining a data scientist’s success. - Linear Algebra and Multivariable Calculus
It may or may not be asked in an interview directly, but at some point, a data scientist may have to build their implementation models in-house. This is particularly true where products that are defined by data can lead to transformation gains for the organization. Data science is a comparatively newer field, and no job descriptions are set in stone. A working knowledge of linear algebra and multi variable calculus can thus come handy when developing out-of-the-box models. Also, an interviewer may stun you with a calculus question. A confident data scientist will tell them to bring it on!
When there are a plethora of courses out there, these questions will help choose the best out of the lot:
- Which course comprehensively covers what I want to learn?
- Which of these courses only rehash topics?
- Which of these courses provide practical experience alongside theoretical knowledge?
- Which courses have the best reviews from students in similar situations?
- Which of these courses is affordable but also worth the money being put into it?
- What sort of reputation does the offering institution have?
- Where have the ex-students of the course been placed within the field?
Conclusion:-
Any person with a structural thought process, good logical thinking skills, conviction towards learning new tools and with a good business perspective can get into the field of data sciences.
It's not exceptional coders or highly knowledgeable people that are required. It is mostly the people who can and are willing to learn new things and cross their comfort zone can get into this domain.
Nothing is impossible here!
Comments
Post a Comment